Zhongbo Yan
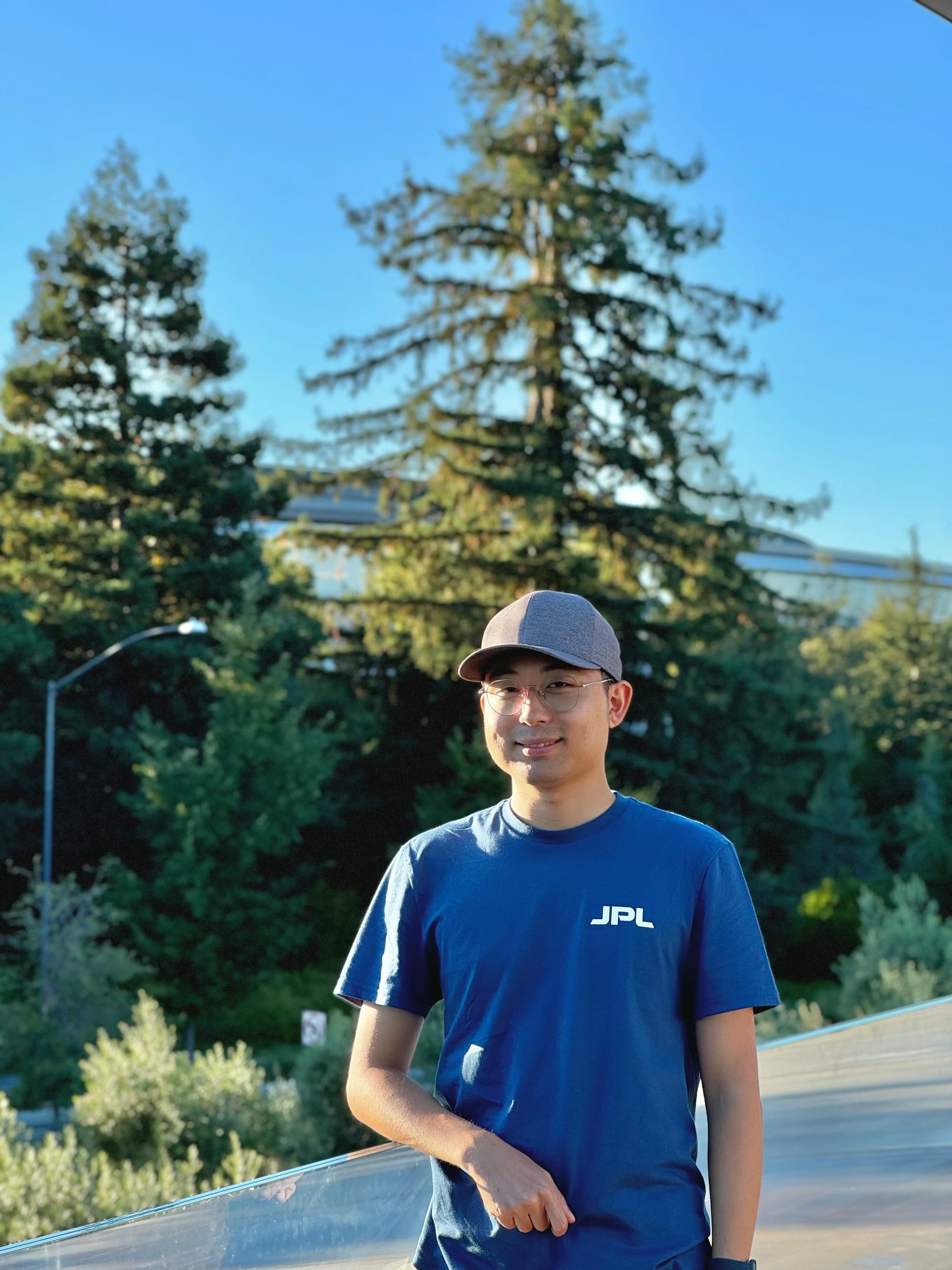
👋 Hello! I’m Zhongbo Yan, a passionate software engineer with interest on cloud computing, machine learning, algorithms, information security, full-stack development, visual designs, and explaining cool stuffs.
I’m currently pursuing my M.S. in Mobile and IoT Engineering at Carnegie Mellon University. I hold a B.S. in Computing from Macao Polytechnic University, where I focused on enterprise information systems.
I have had the privilege of working as a full-stack SDE Intern in Suzhou and Macao. Additionally, I have contributed to academic research, most notably in the field of adversarial data augmentation on knee MRI datasets. My work led to a noticeable improvement in the generalizability of AlexNet on medical MRI datasets, surpassing state-of-the-art models from Stanford University. These findings were published in the IEEE 14th ICSESS.

Feel free to reach out to me through my GitHub and LinkedIn. You can also email me at me at aspires dot cc.
Tech-stacks I used are:
Thank you for visiting my blog! I look forward to connecting with like-minded individuals and exploring new opportunities in the field of software engineering and beyond.